Women in AI Research (WiAIR)
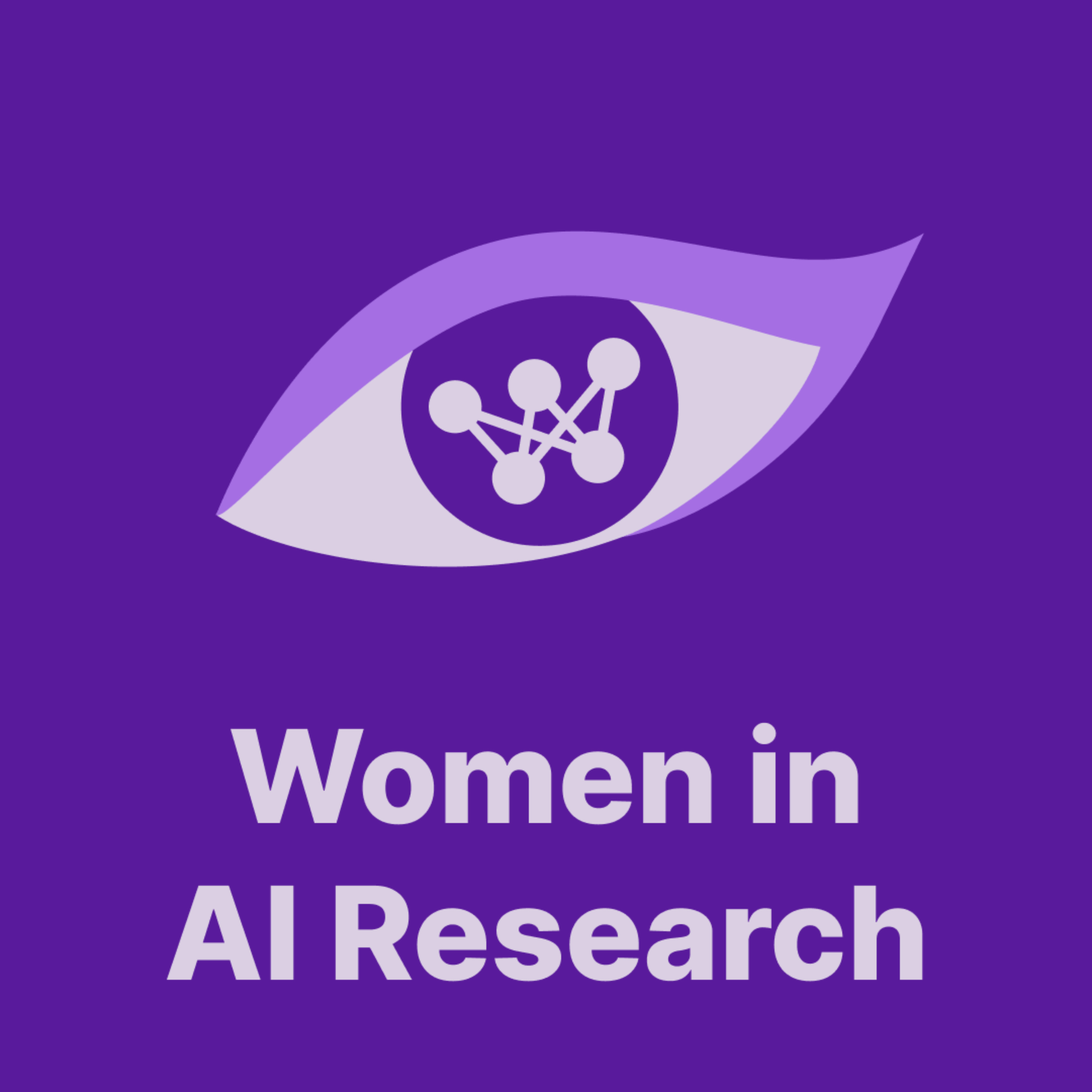
Women in AI Research (WiAIR)
Podcast Description
Women in AI Research (WiAIR) is a podcast dedicated to celebrating the remarkable contributions of female AI researchers from around the globe. Our mission is to challenge the prevailing perception that AI research is predominantly male-driven. Our goal is to empower early career researchers, especially women, to pursue their passion for AI and make an impact in this rapidly growing field. You will learn from women at different career stages, stay updated on the latest research and advancements, and hear powerful stories of overcoming obstacles and breaking stereotypes.
Podcast Insights
Content Themes
The podcast focuses on topics such as bias in AI, the limitations of transformer models, and the personal journeys of women in AI research. Episodes include discussions on the social implications of AI, technical challenges in language models, and the overall impact of diverse voices in the AI field.
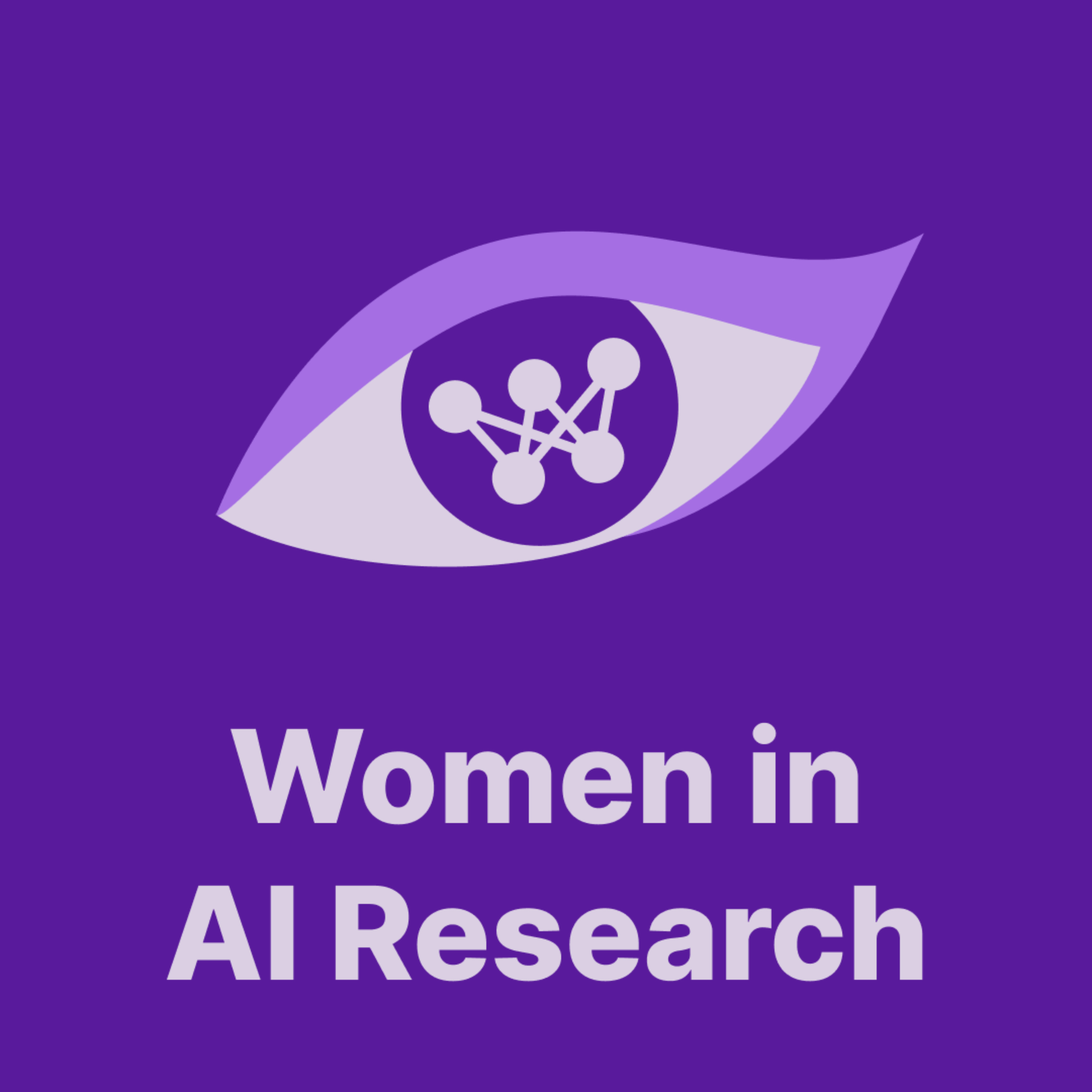
Women in AI Research (WiAIR) is a podcast dedicated to celebrating the remarkable contributions of female AI researchers from around the globe. Our mission is to challenge the prevailing perception that AI research is predominantly male-driven. Our goal is to empower early career researchers, especially women, to pursue their passion for AI and make an impact in this rapidly growing field. You will learn from women at different career stages, stay updated on the latest research and advancements, and hear powerful stories of overcoming obstacles and breaking stereotypes.
Aparna Balagopalan is a PhD student in the Department of Electrical Engineering and Computer Science (EECS) at the Massachusetts Institute of Technology.
In this episode, we present the intersection of AI and healthcare. Aparna shares her research on developing fair, interpretable, and robust models for healthcare applications. We explore the unique challenges of applying AI in medical contexts, including data quality, collaboration with clinicians, and the critical importance of model transparency. The conversation covers both technical innovations and ethical frameworks necessary for responsible AI deployment in healthcare settings.
REFERENCES:
- Aparna's Google Scholar profile
- Machine learning for healthcare that matters: Reorienting from technical novelty to equitable impact
- Judging facts, judging norms: Training machine learning models to judge humans requires a modified approach to labeling data
- LEMoN: Label Error Detection using Multimodal Neighbors
WiAIR website:
Follow us at:
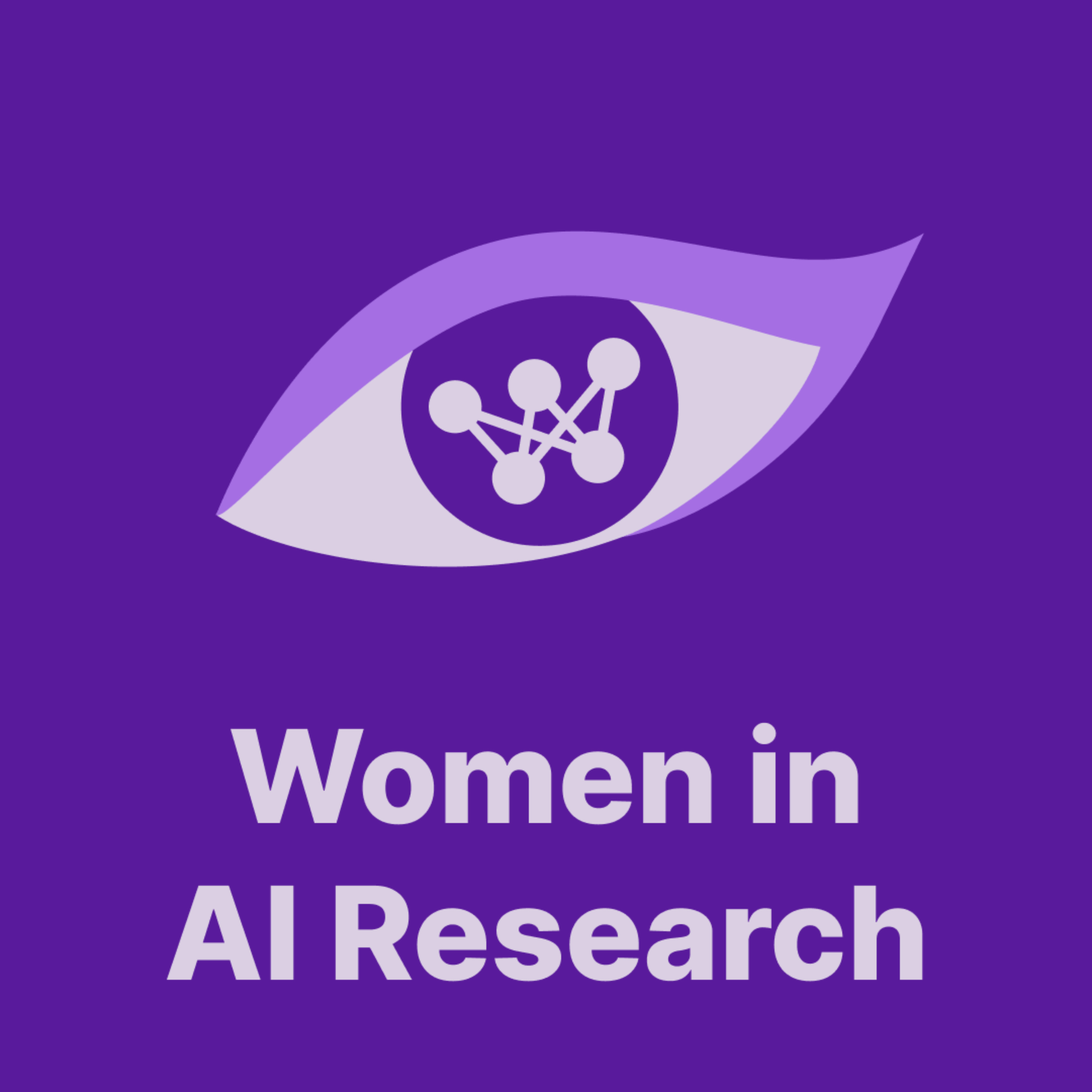
Disclaimer
This podcast’s information is provided for general reference and was obtained from publicly accessible sources. The Podcast Collaborative neither produces nor verifies the content, accuracy, or suitability of this podcast. Views and opinions belong solely to the podcast creators and guests.
For a complete disclaimer, please see our Full Disclaimer on the archive page. The Podcast Collaborative bears no responsibility for the podcast’s themes, language, or overall content. Listener discretion is advised. Read our Terms of Use and Privacy Policy for more details.