Coredump Sessions
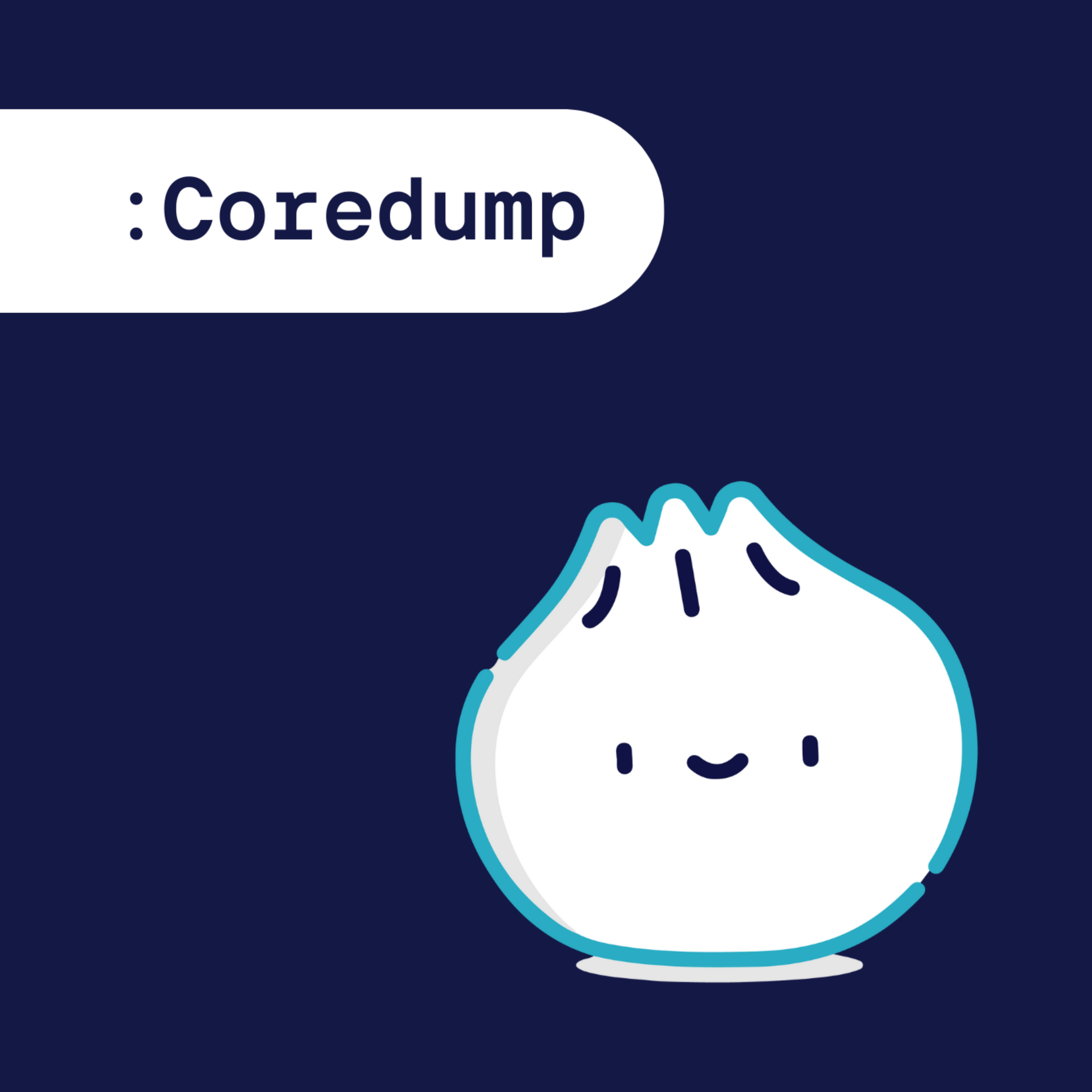
Coredump Sessions
Podcast Description
Coredump Sessions is a podcast for embedded engineers and product teams building connected devices. Hosted by the team at Memfault, each episode features real-world stories and technical deep dives with experts across the embedded systems space.
From Bluetooth pioneers and OTA infrastructure veterans to the engineers who built Pebble, we explore the tools, techniques, and tradeoffs that power reliable, scalable devices. If you're building or debugging hardware, this is your go-to for embedded insights.
Podcast Insights
Content Themes
The podcast focuses on various aspects of embedded engineering, featuring topics like open-source firmware, Bluetooth technology, and device scalability, with episodes such as the discussion around the open-sourcing of Pebble OS and its implications for developers and the industry.
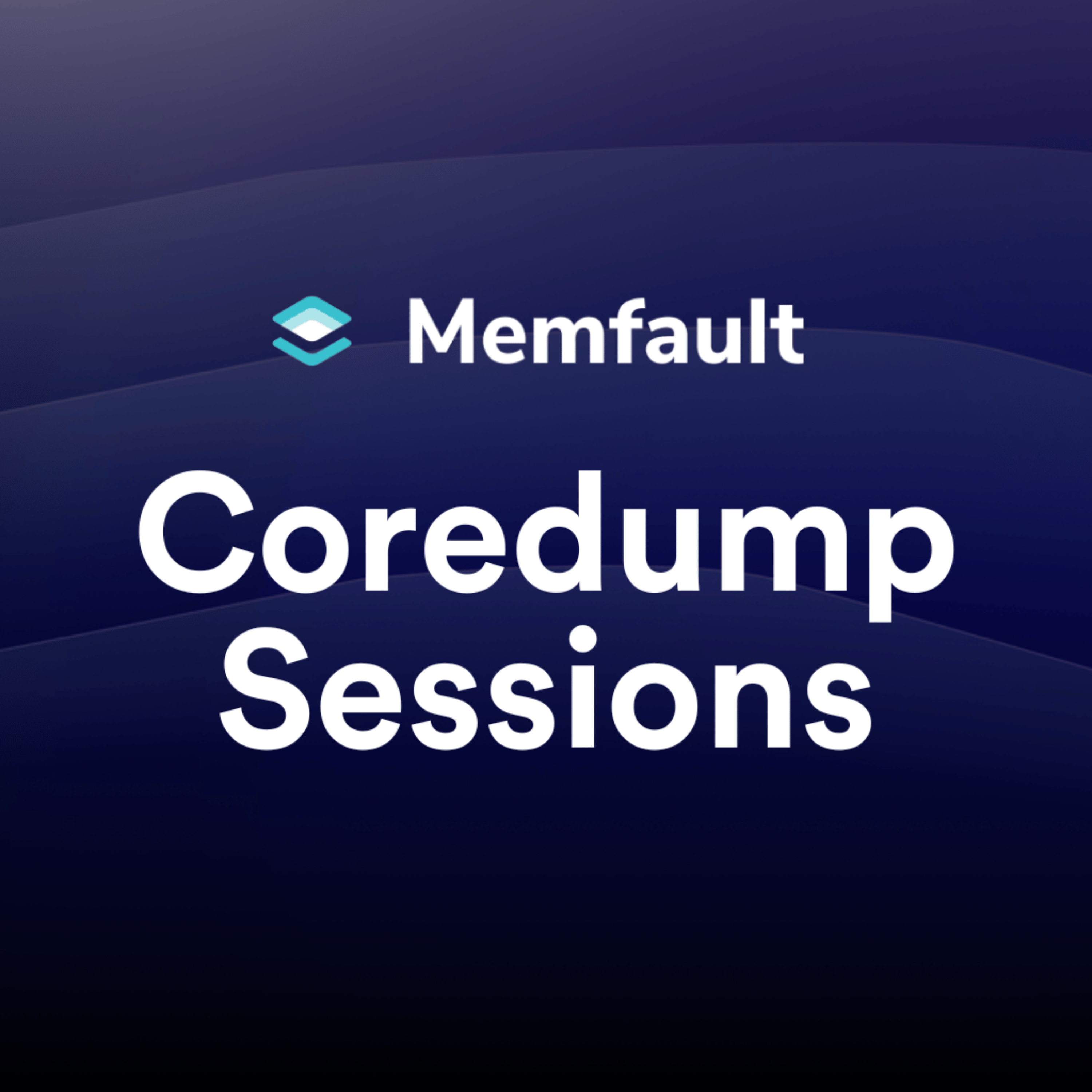
Coredump Sessions is a podcast for embedded engineers and product teams building connected devices. Hosted by the team at Memfault, each episode features real-world stories and technical deep dives with experts across the embedded systems space.
From Bluetooth pioneers and OTA infrastructure veterans to the engineers who built Pebble, we explore the tools, techniques, and tradeoffs that power reliable, scalable devices. If you’re building or debugging hardware, this is your go-to for embedded insights.
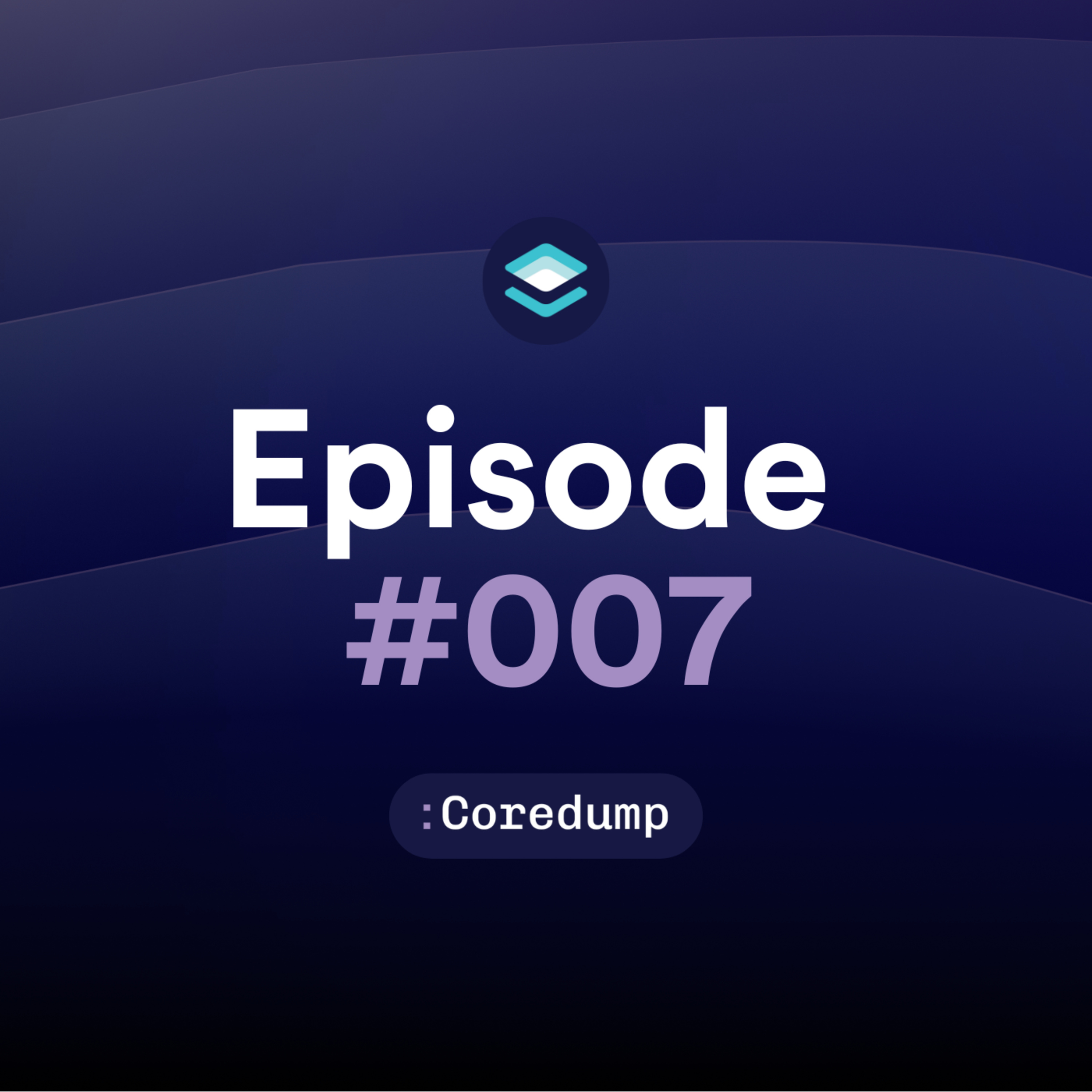
In today's Coredump Session, we dive into a wide-ranging conversation about the intersection of AI, open source, and embedded systems with the teams from Memfault and Goliath. From the evolution of AI at the edge to the emerging role of large language models (LLMs) in firmware development, the panel explores where innovation is happening today — and where expectations still outpace reality. Listen in as they untangle the practical, the possible, and the hype shaping the future of IoT devices.
Speakers:
- François Baldassari: CEO & Founder, Memfault
- Thomas Sarlandie: Field CTO, Memfault
- Jonathan Beri: CEO & Founder, Golioth
- Dan Mangum: CTO, Golioth
Key Takeaways:
- AI has been quietly powering embedded devices for years, especially in edge applications like voice recognition and computer vision.
- The biggest gains in IoT today often come from cloud-based AI analytics, not necessarily from AI models running directly on devices.
- LLMs are reshaping firmware development workflows but are not yet widely adopted for production-grade embedded codebases.
- Use cases like audio and video processing have seen the fastest real-world adoption of AI at the edge.
- Caution is warranted when integrating AI into safety-critical systems, where determinism is crucial.
- Cloud-to-device AI models are becoming the go-to for fleet operations, anomaly detection, and predictive maintenance.
- Many promising LLM-based consumer products struggle because hardware constraints and cloud dependence create friction.
- The future of embedded AI may lie in hybrid architectures that balance on-device intelligence with cloud support.
Chapters:
00:00 Episode Teasers & Welcome
01:10 Meet the Panel: Memfault x Golioth
02:56 Why AI at the Edge Isn’t Actually New
05:33 The Real Use Cases for AI in Embedded Devices
08:07 How Much Chaos Are You Willing to Introduce?
11:19 Edge AI vs. Cloud AI: Where It’s Working Today
13:50 LLMs in Embedded: Promise vs. Reality
17:16 Why Hardware Can’t Keep Up with AI’s Pace
20:15 Building Unique Models When Public Datasets Fail
36:14 Open Source’s Big Moment (and What Comes Next)
42:49 Will AI Kill Open Source Contributions?
49:30 How AI Could Change Software Supply Chains
52:24 How to Stay Relevant as an Engineer in the AI Era
Follow Memfault
Other ways to listen:
Disclaimer
This podcast’s information is provided for general reference and was obtained from publicly accessible sources. The Podcast Collaborative neither produces nor verifies the content, accuracy, or suitability of this podcast. Views and opinions belong solely to the podcast creators and guests.
For a complete disclaimer, please see our Full Disclaimer on the archive page. The Podcast Collaborative bears no responsibility for the podcast’s themes, language, or overall content. Listener discretion is advised. Read our Terms of Use and Privacy Policy for more details.